Your Journey to Master Deep Learning Starts Here
Deep learning algorithms are revolutionizing data science industry and disrupting several domains. From computer vision applications to natural language processing (NLP) use cases - every field is benefitting from use of Deep Learning models.
This course starts by assuming no knowledge about Neural Networks and Deep Learning and introduces these subjects to the student one by one.
The course helps you build a deep as well as intuitive understanding of what is Deep Learning, where can Deep Learning Models be applied and then helps you solve several real life problems using Keras and PyTorch frameworks.
Tools and Techniques covered in Fundamentals of Deep Learning Course
Fundamentals of Deep Learning covers every tool a data scientist needs to build Deep Learning models
-
Convolution Neural Networks (CNN)
-
Recurrent Neural Networks (RNNs)
-
Advanced Sequence Models including LSTM & GRU
-
Transfer Learning
What will you learn in the Fundamentals of Deep Learning course?
- 258 Lesssons
- 5 Real Life Projects from Data Science Industry
Tools Covered in the Fundamentals of Deep Learning Course
Key takeaways from Fundamentals of Deep Learning Course
These deep learning algorithms are powered by techniques like Convolutional Neural Networks (CNN), Recurrent Neural Networks (RNN), Long Short Term Memory (LSTM), etc.
If you’re a beginner in deep learning, or you are looking to hone your existing deep learning and neural network skills, you must have asked these questions:
What is deep learning?
What are the different types of neural networks?
What part do these neural networks play in the deep learning space?
When should you choose convolutional neural networks (CNN) over recurrent neural networks (RNN) - and vice versa?
How can you build deep learning models in Python?
What is forward propagation? And what is backpropagation?
What are the different loss functions in deep learning?
What are the different deep learning frameworks?
TensorFlow vs. PyTorch vs. Keras - which deep learning framework should you choose?
The course is designed to answer all the above questions in an applied first methodology.
Projects of Fundamentals of Deep Learning
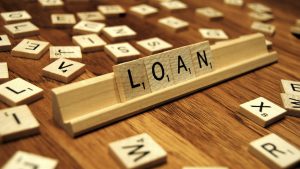
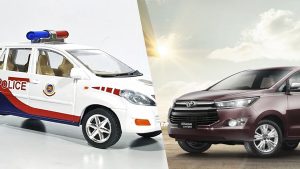
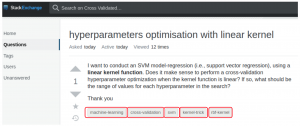
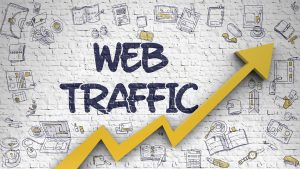
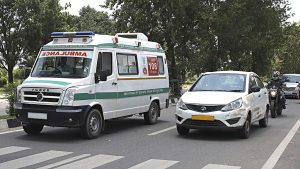
Course curriculum
-
1
Introduction to Deep Learning
- What is Deep Learning? FREE PREVIEW
- Difference b/w Deep Learning and Machine Learning FREE PREVIEW
- Quiz: Difference between Machine Learning and Deep Learning
- Why Deep Learning is so popular? FREE PREVIEW
- Quiz: Why Deep learning is so popular?
-
2
Getting ready for the course
- Hardware for Computations in Deep Learning FREE PREVIEW
- Setting up your system
- Introduction to Google Colab FREE PREVIEW
- Understanding Google Colab Interface FREE PREVIEW
- Pre-requisites for Deep Learning
-
3
Introduction to the course
- Structure of course FREE PREVIEW
- Instructor introduction FREE PREVIEW
- Course Handouts
-
4
Introduction to Neural Network
- Perceptron FREE PREVIEW
- Quiz - Perceptron
- Weights in Perceptron FREE PREVIEW
- Quiz - Weights in Perceptron
- Multi Layer Perceptron FREE PREVIEW
- Quiz - Multi Layer Perceptron
- Visualizing the neural network FREE PREVIEW
- Understanding Decision Boundary
- Quiz: Understanding the decision boundary
- Quiz - Visualizing the neural network
- Forward and Backward Prop Intuition
- Quiz - Forward and Backward Prop Intuition
- Gradient Descent Algorithm
- Quiz - Gradient Descent Algorithm
-
5
Forward and Backward Propagation
- Understanding Forward Propagation Mathematically
- Quiz - Understanding Forward Propagation Mathematically
- Understanding Backward Propagation Mathematically
- Quiz - Understanding Backward Propagation Mathematically
- Backward Propagation: Matrix Form
- Why Numpy?
- Quiz: Why Numpy?
- Neural Network From scratch Using Numpy
- Quiz: Implementation of Neural Network
- Forward Propagation (using Numpy)
- Backward Propagation (using Numpy)
- Training network (using Numpy)
-
6
Activation Functions
- Why do we need activation functions?
- Quiz - Why do need activation functions
- Linear Activation Function
- Quiz - Linear Activation Function
- Sigmoid and tanh
- Quiz - Sigmoid and tanh
- ReLU and Leaky ReLU
- Quiz - ReLU and LeakyReLU
- Softmax
- Quiz - Softmax
- Tips to selecting right Activation Function
- Quiz: Tips to selecting right activation function
-
7
Optimizers
- Variants of Gradient Descent
- Quiz - Variants of Gradient Descent
- Problems with Gradient Descent
- Quiz - Problems with Gradient Descent
- RMSProp
- Quiz - RMSProp
- Adam
- Quiz: Adam
- Expert Talk: Vijay Gabale
-
8
Loss Function
- Introduction to loss function
- Quiz - Introduction to Loss Function
- Binary and Categorical Cross entropy / log loss
- Quiz - Binary and Categorical cross entropy / log loss
-
9
NN on structured Data
- Overview of Deep Learning Frameworks
- Quiz - Overview of deep learning frameworks
- Understanding important Keras modules
- Quiz: Understanding important Keras Modules
- Understanding the problem statement: Loan Prediction
- Data Preprocessing: Loan Prediction
- Quiz - Data Preprocessing: Loan Prediction
- Steps to solve Loan Prediction Challenge
- Loading loan prediction dataset
- Quiz: Understanding the problem statement : loan prediction
- Defining the Model Architecture for loan prediction problem
- Quiz: Defining the model architecture for loan prediction problem
- Training and Evaluating model on Loan Prediction Challenge
- Quiz - Training and Evaluating model on Loan Prediction Challenge
-
10
Assignment: Big Mart Sales Prediction
- Assignment: Big Mart Sales Prediction
-
11
Functional API in Keras for Deep Learning
- Functional API for Deep Learning
- Quiz - Functional API for Deep Learning
- Solving Loan Prediction Using Functional API in Keras
- Building a custom Model Using Functional API in keras
- Quiz - Building a Model Using Functional API
-
12
Getting started with image data
- How are images stored?
- Quiz - How are images stored
- Different Image Formats
- Quiz - Different Image formats
- Reading and stacking Images
- Quiz: Reading and stacking images
- Converting images into different formats
- Quiz - Converting image into different formats
- Extracting edges from images
- Quiz - Extracting edges from images
- Implementation: Extracting edges from images
- Quiz - Extracting edges implementation
-
13
Solving Image Classification Using Keras
- Project: Image Classification "Emergency Vs Non-Emergency Vehicle"
- Notebook: Neural Network in Keras and Hyperparameter Tuning
- Neural Network in Keras
- Quiz: Neural network in keras
- Hyperparameter Tuning for MLP in Keras
- Quiz: Hyperparameter tuning for MLP in keras
-
14
Assignment: Gender Classification
- Assignment: Gender Classification
-
15
Improving your Deep Learning Model
- Early stopping
- Early stopping: Implementation
- Quiz - Early stopping: Implementation
- Dropout
- Dropout: Implementation
- Quiz - Dropout: Implementation
- Vanishing and Exploding gradients
- Quiz: Vanishing and exploding gradients
- Vanishing and Exploding gradients: Implementation
- Quiz - Vanishing and exploding gradient: Implementation
- Weights Initialization Techniques
- Quiz: Weight Initialization techniques
- Implementing different weight initializing techniques
- BatchNorm
- Quiz: Batch Normalization
- BatchNorm: Implementation
- Advantages of Batch Normalization
- Quiz - Advantages of Batch Normalization
- Image Augmentation Techniques
- Image Augmentation Techniques: Implementation
- Quiz: Image Augmentation: Implementation
- Image Augmentation on Emergency-non emergency dataset
- Image Generator and Fit Generator
- Quiz: Image Generator and Fit generator
- Assignment: Gender Classification
- Model Checkpointing
- Quiz: Model Checkpointing
- Implementing model checkpointing
-
16
Introduction to Convolutional Neural Network and Implementation
- Why do we need CNN?
- Quiz - Why do we Need CNN
- How filters work?
- Quiz - How filters work?
- Filters in CNN
- Quiz - Filters in CNN
- Parameter Sharing and Local Connectivity in CNN
- Quiz - Parameter Sharing and Local Connectivity
- CNN Architecture
- Quiz - CNN Architecture
- Pooling
- Quiz - Pooling
- CNN Forward Propagation
- Quiz - CNN Forward Propagation
- CNN Backward Propagation
- Quiz - CNN Backward propagation
- CNN Backprop : Matrix Form (Optional)
- Convolutional Neural Network in Keras
- Quiz - CNN in keras
- Hyperparameter Tuning for CNN in keras
- Quiz - Hyperparameter Tuning for CNN in keras
- Assignment: Gender Classification
- Model Checkpointing in CNN
-
17
Introduction to Transfer Learning
- Introduction to Transfer Learning FREE PREVIEW
- How to select right pre-trained model?
- Exercise : How to select right pre-trained model?
- Steps to solve emergency vs non emergency vehicle classification challenge using transfer learning
- Exercise : Steps to build the model using transfer learning
- Solving the challenge using transfer learning (Notebook)
- Solving the challenge using Transfer Learning (Part I)
- Exercise: Solving the challenge using Transfer Learning
- Solving the challenge using Transfer Learning (Part II)
- Exercise: Solving the challenge using Transfer Learning -II
- Solving the challenge using Transfer Learning (Part III)
- Exercise : Solving the challenge using transfer learning - III
- Different fine tuning techniques
- Transfer Learning: Using the architecture of the pre-trained model
- Quiz: Transfer learning Using architecture of pretrained model
- Transfer Learning: Freezing some layers and training others
- Advantages of transfer learning
- Assignment: Gender Classification
-
18
CNN Visualization
- Introduction to Neural Network Visualization
- Quiz - Introduction to Neural Network Visualization
- How can we Interpret a Neural Network?
- Quiz: How can we interpret a Neural network
- Setting up the System
- Attempt 1: Understand the model architecture
- Quiz - Attempt 1: Understand the model architecture
- Attempt 2: Visualize the filters / weights
- Quiz - Attempt 2: Visualize the filters / weights
- Attempt 3: Extract the output of intermediate layers
- Quiz - Attempt 3: Extract the output of intermediate layers
- Attempt 4: Locate important parts of the image
- Quiz - Attempt 4: Locate important parts of the image
-
19
Real World Use Cases of Deep Learning
- Object Detection, segmentation, image generation
- Quiz - Object Detection, Segmentation, image generation
- Sequential Modeling
- Quiz - Sequential Modeling
-
20
Working with Text Data
- Getting Started with Text Data
- Quiz - Getting Started with Text Data
- Introduction to Text Preprocessing
- Quiz - Intro to Text-Preprocessing
- Regular Expressions in Action - I
- Regular Expressions in Action - II
- Regular Expressions in Action - III
- Quiz - Regular Expressions in Action
- Understanding Text Representation
- Quiz: Understanding text representation
- Introduction to One-Hot-Encoding
- Quiz - Introduction to One-Hot-Encoding
- Implementation: One-Hot-Encoding
- Quiz: Implementation: One hot encoding
- Limitations of One-Hot-Encoded Vectors
- Quiz - Limitations of One-Hot-Encoded Vectors
- Word Embeddings
- Implementation: Word Embeddings
- Quiz - Word Embeddings
-
21
Getting Started with Recurrent Neural Networks (RNN)
- Journey So Far
- Introduction to RNN
- Quiz - Introduction to RNN
- Forward Propagation in RNN
- Quiz - Forward Propagation in RNN
- Backward Propagation Through Time - Part 1
- Backward Propagation Through Time - Part 2
- Backward Propagation Through Time - Part 3
- Quiz - BPTT
-
22
Project - Building an Auto-Tagging System
- Overview of Auto-Tagging System
- Quiz - Overview of Auto-Tagging System
- Understanding the Dataset
- Merging Different Datasets
- Quiz: Merging different dataset
- Dataset Preparation
- Quiz: Dataset preparation
- Model Building and Tags Prediction
- Quiz - Model Building and Tags Prediction
-
23
Advanced Sequence Models - LSTM & GRU
- Shortcomings of RNN
- Quiz - Shortcomings of RNN
- What is Long Short Term Memory (LSTM) Network?
- Quiz - What is Long Short Term Memory (LSTM) Network?
- What is Gated Recurrent Unit (GRU) Network?
- Quiz - What is Gated Recurrent Unit (GRU) Network?
- Solving Auto-Tagging Problem using LSTM and GRU
- How to use CNN for Text Data?
- Quiz - CNN for Text Data
- Solving Auto-Tagging Problem using CNN
- Quiz: Solving Auto-tagging problem using CNN
-
24
Assignment: Identify the Sentiments
- Assignment: Identify the Sentiments
-
25
Project - Web Traffic Forecasting
- Overview of Web Traffic Forecasting Problem
- Quiz - Overview of Web Traffic Forecasting Problem
- Data Exploration and Pre-processing
- Quiz - Data Exploration and Pre-processing
- Model Building and Forecasting
-
26
Project - Emergency vs Non Emergency Vehicle Sound Classification
- Introduction to Audio Data
- Quiz: Introduction to Audio data
- Understanding the Audio Classification Problem
- Audio Data Preparation
- Quiz: Audio data preparation
- Audio Classification using Time Domain Features
- Quiz: Audio Classification using Time Domain features
- Audio Classification using Spectrogram features
- Quiz: Audio classification using Spectrogram features
-
27
Assignment: Urban Sound Classification
- Assignment: Urban Sound Classification
-
28
Unsupervised Deep Learning
- Recap of Previous Modules
- Introduction to Unsupervised Learning
- Quiz - Introduction to Unsupervised Learning
- How to Solve Unsupervised Learning Problems?
- Introduction to Autoencoders
- Quiz - Introduction to Autoencoders
- Photo Gallery Organizing using Autoencoders
- Dataset: Photo Gallery Organization
- Implementation: Photo Gallery Organization
- Quiz: Implementation: Photo gallery Organization
- Unsupervised Deep Learning - Problems and Research
-
29
Assignment: Image Denoising
- Assignment: Image Denoising
- Image Denoising Dataset
-
30
Introduction to PyTorch
- Overview of the Module
- Introduction to PyTorch and tensors
- Quiz: Introduction to PyTorch and tensors
- Mathematical and matrix operations in PyTorch - Part I
- Mathematical and matrix operations in PyTorch - Part II
- Mathematical and matrix operations in PyTorch - Part III
- Quiz: Mathematical and matrix operations in Pytorch
- Neural Network from scratch in PyTorch - Part I
- Neural Network from scratch in PyTorch - Part II
- Neural Network from scratch in PyTorch - Part III
- Quiz: Neural networks from scratch in PyTorch
- Important PyTorch modules (Autograd, nn, optim, etc.)
- Quiz: Important PyTorch modules
- Solving image classification problem using MLP in PyTorch - Part I
- Solving image classification problem using MLP in PyTorch - Part II
- Solving image classification problem using MLP in PyTorch - Part III
- Solving image classification problem using MLP in PyTorch - Part IV
- Quiz: Solving Image classification problem using MLP in PyTorch
- Convolutional Neural Network in PyTorch
- Quiz: Convolutional neural network in PyTorch
- Hyperparameter Tuning of CNN
- Quiz: Hyperparameter tuning in CNN
- CNN Improvements
- Quiz: CNN Improvements
- Transfer Learning in PyTorch - Part I
- Transfer Learning in PyTorch - Part II
- Quiz: Transfer Learning in PyTorch
- Working with text data in pytorch
- Quiz: Working with Text data in PyTorch
- Text preprocessing in pytorch
- Quiz: Text Preprocessing in PyTorch
- Building an RNN model in PyTorch
- Quiz: Building RNN model in PyTorch
- Evaluating RNN model
- Building and evaluating LSTM in PyTorch
- Quiz: Building and evaluating LSTM in PyTorch
- Autoencoders in PyTorch
- Quiz: Auto-encoder in PyTorch
-
31
What's Next?
- Where to go from here?
Certificate of Completion

Instructor(s)
-
Aishwarya Singh
Aishwarya is currently working as a Data Scientist at Analytics Vidhya. She is one of the primary content curators and an instructor for Analytics Vidhya’s most popular course – Applied Machine Learning. She is also an avid reader and blogger who loves exploring the endless world of data science and artificial intelligence. She has written over 70 articles in recent years on various machine learning and deep learning topics and applications. -
Prateek Joshi
Prateek is a Data Scientist at Analytics Vidhya. He has a multidisciplinary academic background and rich experience in BFSI and E-Learning industries. Prateek's strengths include expertise in Natural Language Processing (NLP) and Machine Learning. He is well versed in Python, R and most of the libraries and frameworks around machine learning and NLP. He has taken various trainings around NLP and Data Science and he is also a course instructor and content creator at Analytics Vidhya. -
Pulkit Sharma
Pulkit is a Data Scientist at Analytics Vidhya. His research area lies in the field of Computer Vision and Deep Learning. He has been working on various projects related to images and videos for the past few years. He is comfortable with Python, Keras, PyTorch and has done multiple projects using these frameworks and tools. Some of his key projects include Crowd Counting, Estimating the Screen Time in videos, Object Detection, and Image Segmentation. He is one of the primary content curators for Analytics Vidhya’s courses, such as the Computer Vision using Deep Learning and Applied Machine Learning. He is also an avid blogger and has written multiple detailed and in-depth guides on various computer vision topics and applications, ranging from Image Classification to Object Detection and Image Segmentation.
Here's what our students have to say
-
Loved the Course!
Umang Verma
Very well explained! Deep Learning concepts were explained from scratch and practical application is discussed throughout the course!
Very well explained! Deep Learning concepts were explained from scratch and practical application is discussed throughout the course!
Read Less -
FAQ
Common Questions Answered about the Fundamentals of Deep Learning course
-
Who should take the Fundamentals of Deep Learning course?
This course is for beginners in deep learning. You don’t need to know anything about neural networks - we’ll cover all of that inside the course!
-
How long would I have access to the “Fundamentals of Deep Learning” course?
Once you register, you will have 6 months to complete the course.
-
How much effort will this course take?
We suggest spending 8-10 hours per week to complete the “Fundamentals of Deep Learning” course and apply your learning on real-world projects. The time taken on projects varies from person to person.
-
What kind of deep learning projects can I take up?
We have provided plenty of real-world deep learning projects in the course, including object detection, image classification, among others.
-
What is the fee for this course?
The fee for this course is Rs. 7,999.
-
Can I download videos from this course?
We regularly update the "Fundamentals of Deep Learning" course and hence do not allow for videos to be downloaded.
-
Which programming language is used in this course?
Deep learning using Python - that’s the idea behind this course. Python is the most popular language for deep learning and you’ll see why when you take the course!
Customer Support for our Courses & Programs
We are there for your support when you need!
-
Phone - 10 AM - 6 PM (IST) on Weekdays (Mon - Fri) on +91-8368808185
-
Email [email protected] (revert in 1 working day)
-
Discussion Forum - answer in 1 working day